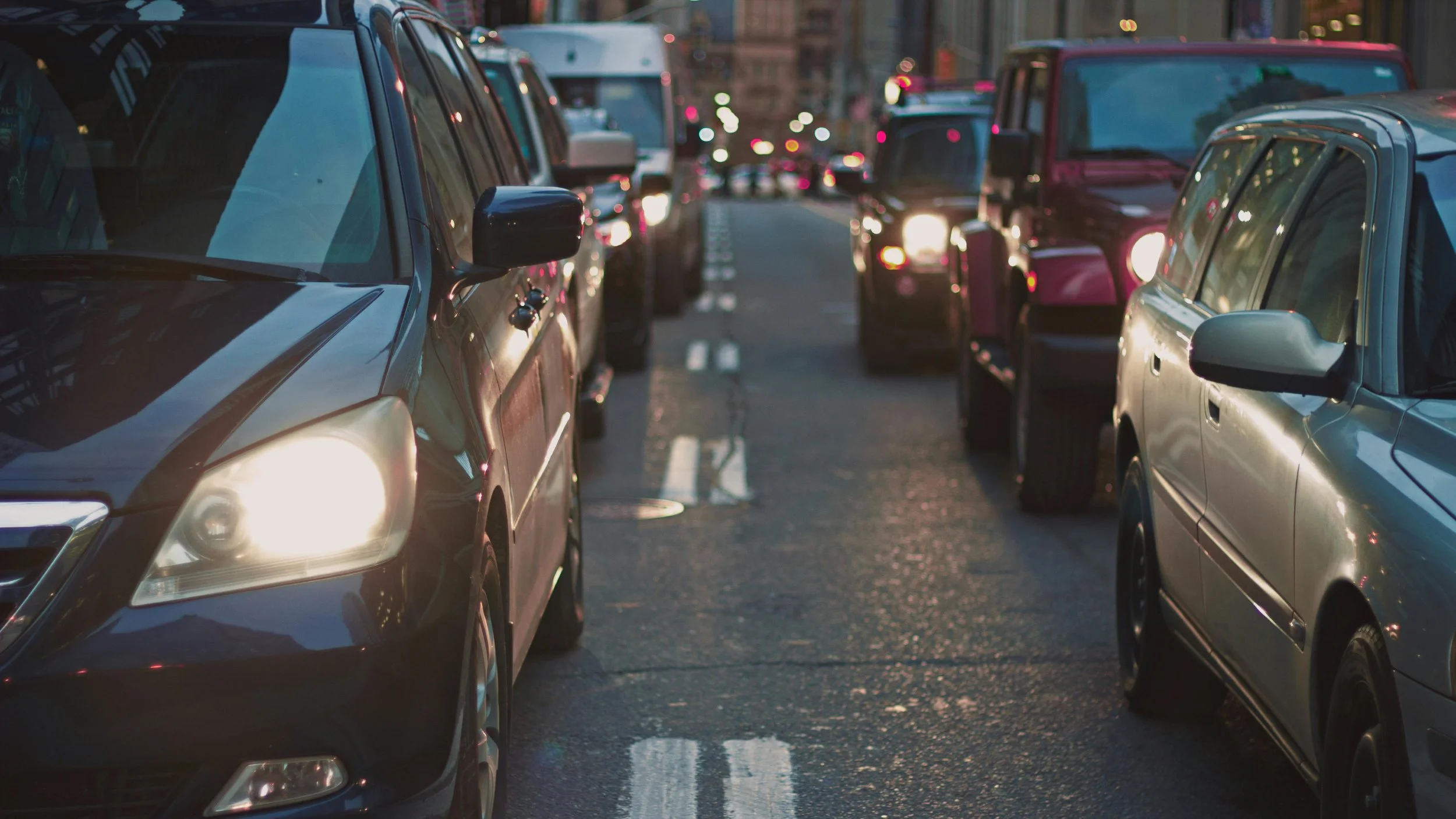
Traffic Behavior Recognition from Traffic Videos under Occlusion Condition: A Kalman Filter Approach

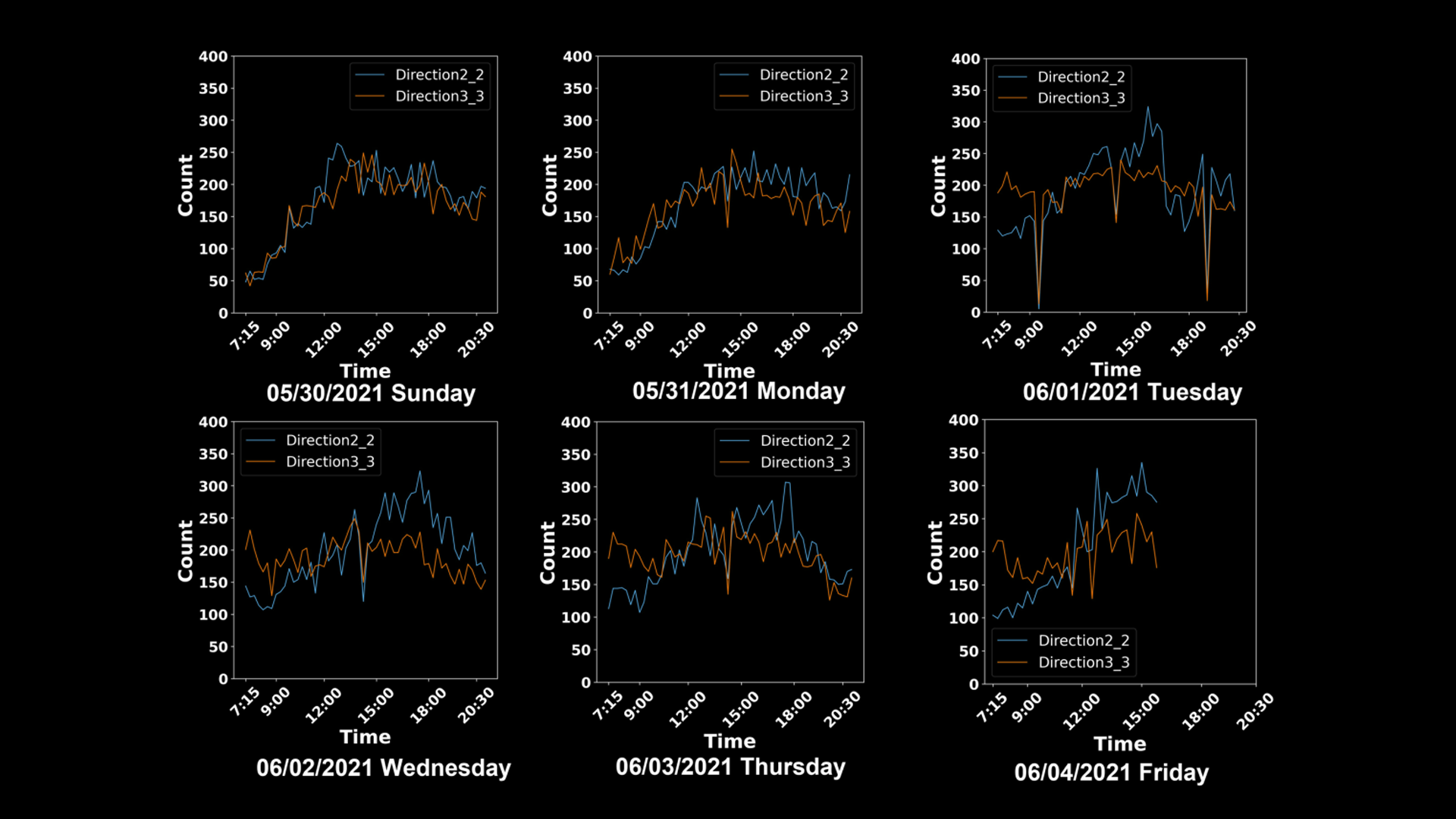
Abstract
Real-time traffic data is crucial for adaptive traffic light control systems. Traditional sensors like infrared radiation and GPS lack detail. Surveillance cameras offer potential for detailed traffic analysis. This study utilizes a You Only Look Once (YOLO) algorithm for vehicle detection and tracking in traffic videos, aided by a predefined road mask. A Kalman filter predicts vehicle speed and location, even with background occlusion. Results show accurate traffic flow and turning event detection with a root mean square error (RMSE) of 10. The Kalman filter, combined with an Intersection over Union (IOU)-based tracker, performs well under background occlusion. The algorithm operates effectively under various optical conditions, though adverse weather and nighttime may affect detection in distant areas. Extracted traffic flow data provides insights for single intersection control and broader road network management. Temporal patterns in traffic flow offer potential for predictive control, enhancing traffic light efficiency.
Team
Junfeng Jiao Huihai Wang