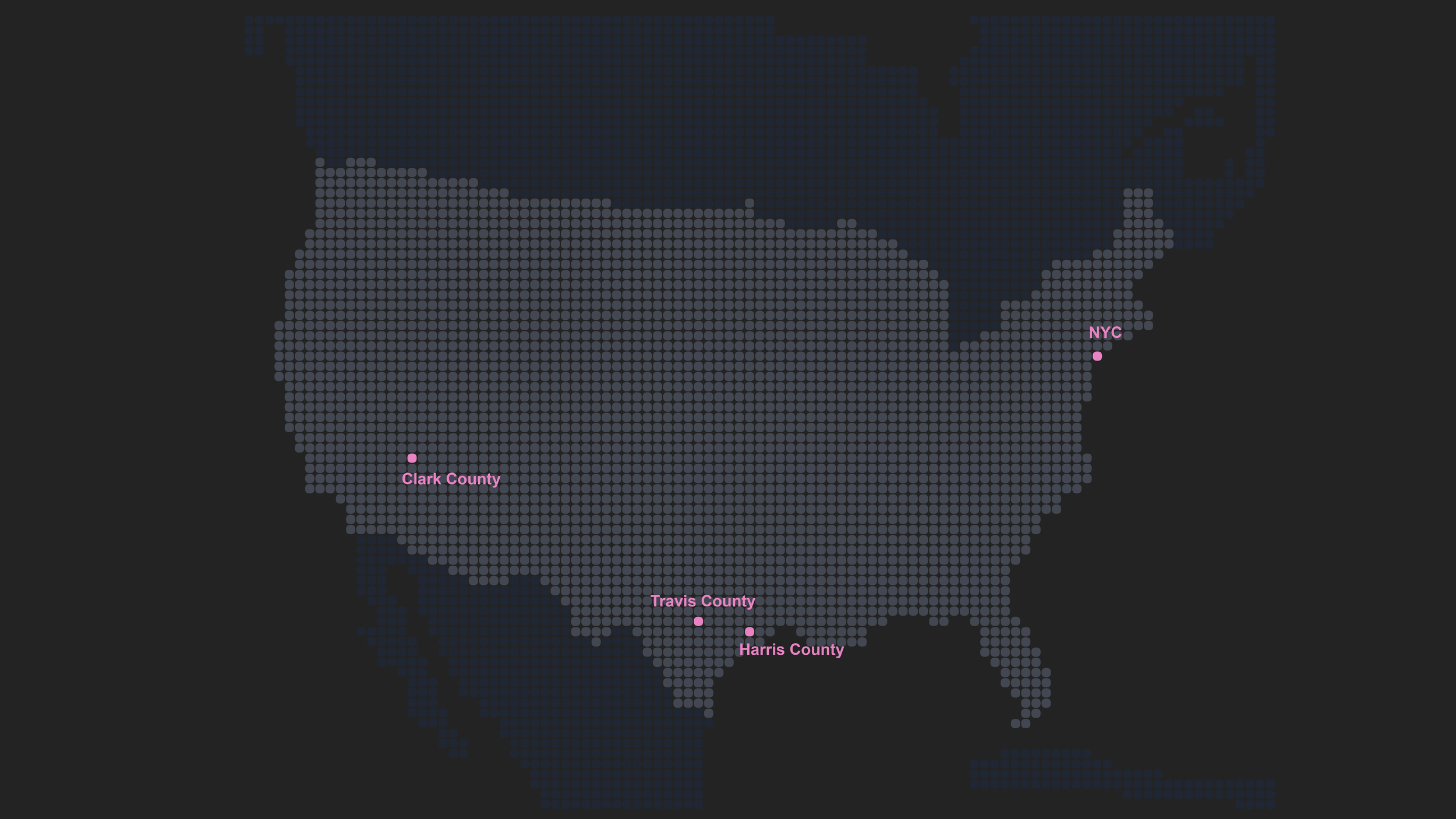
Temporal Disparities in COVID-19: U.S. Insights with OLS, GWR, and Random Forest
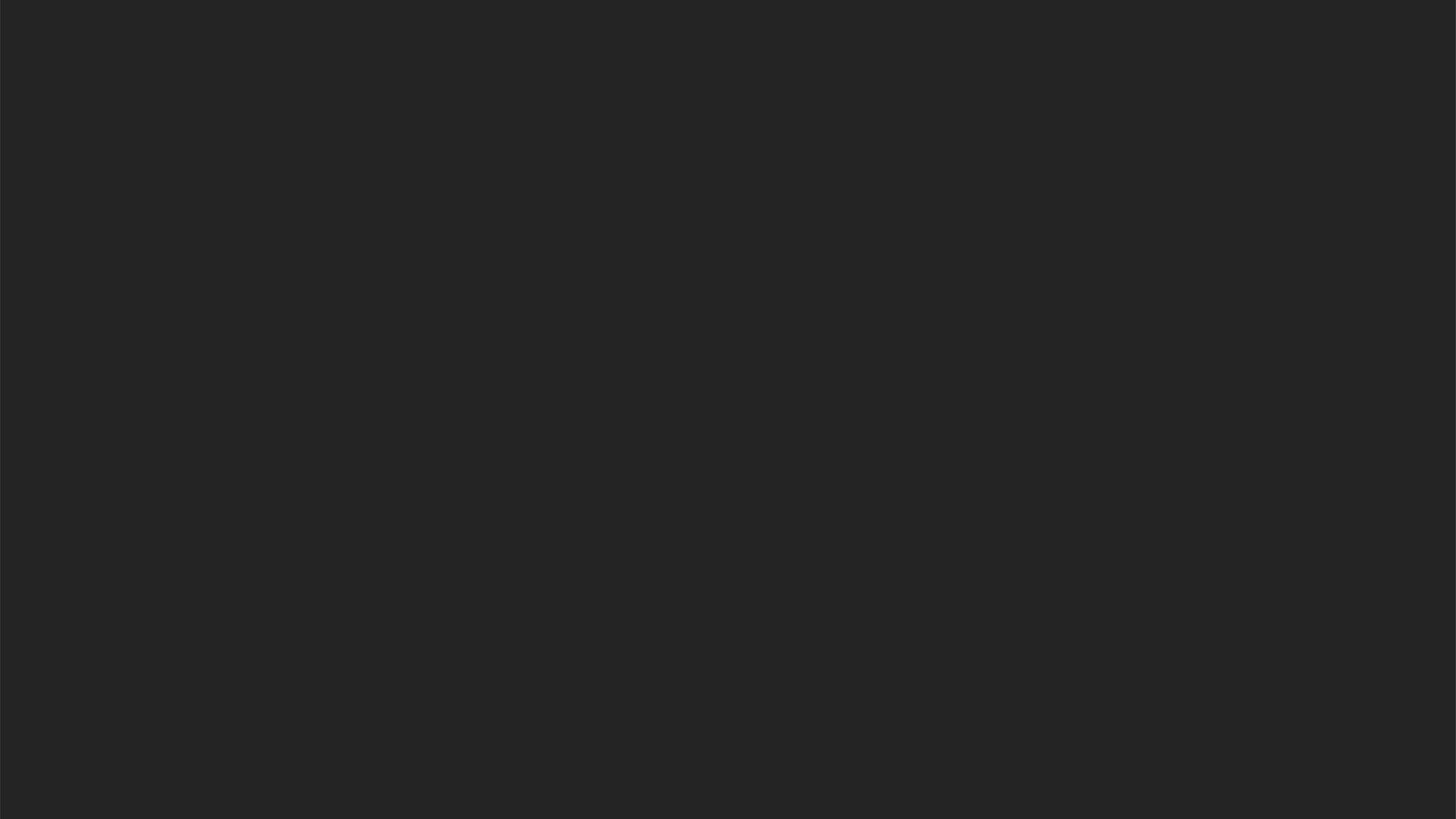
This study explores economic-demographic disparities in COVID-19 infections across varied population densities in the United States, employing OLS, GWR, and random forest models on zip code-level data from four regions. Significant disparities were found, with high-risk areas among disadvantaged groups showing varying infection risks across pandemic periods. Results inform smarter public health planning, guiding comprehensive resource allocation during crises.

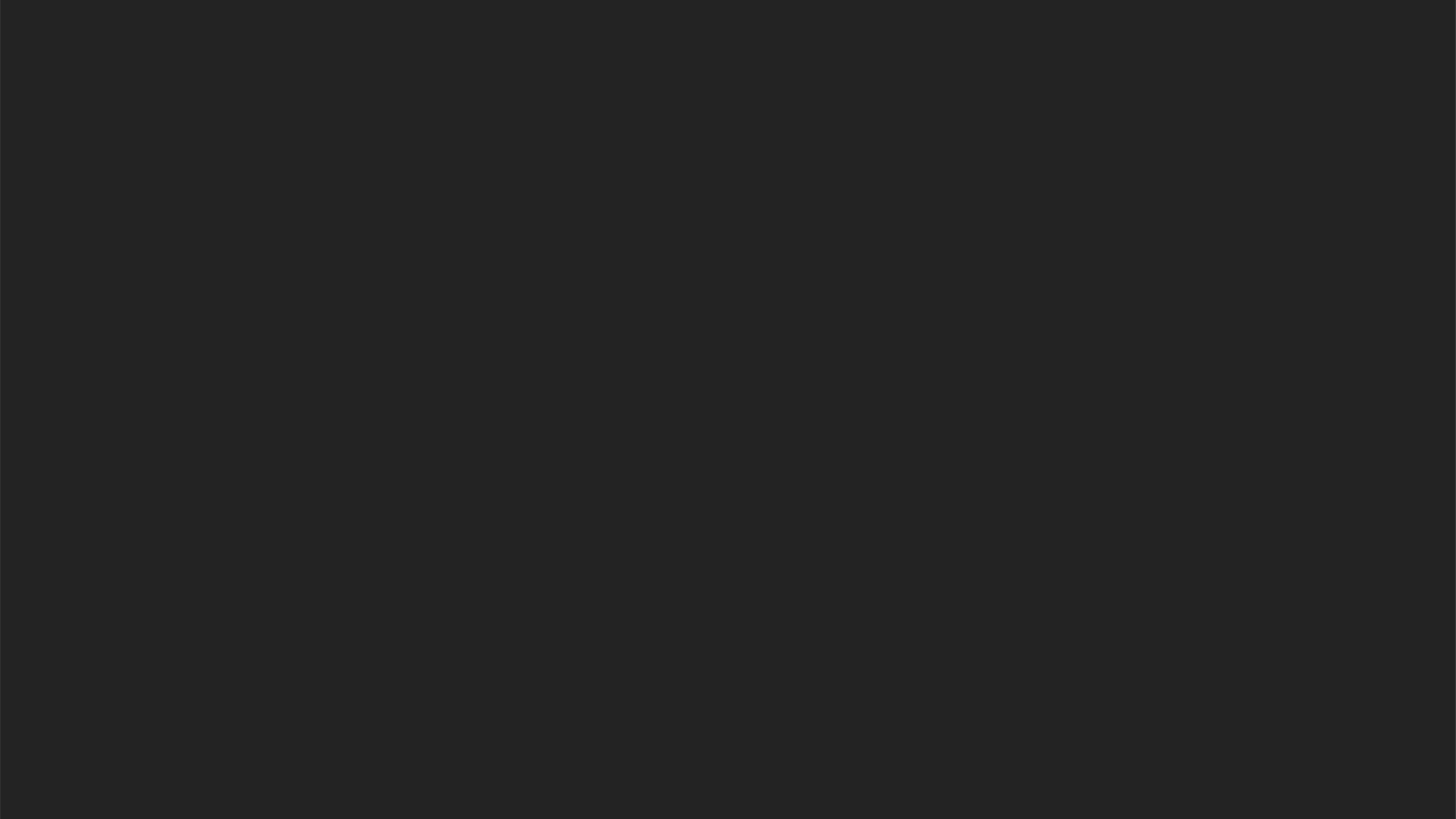