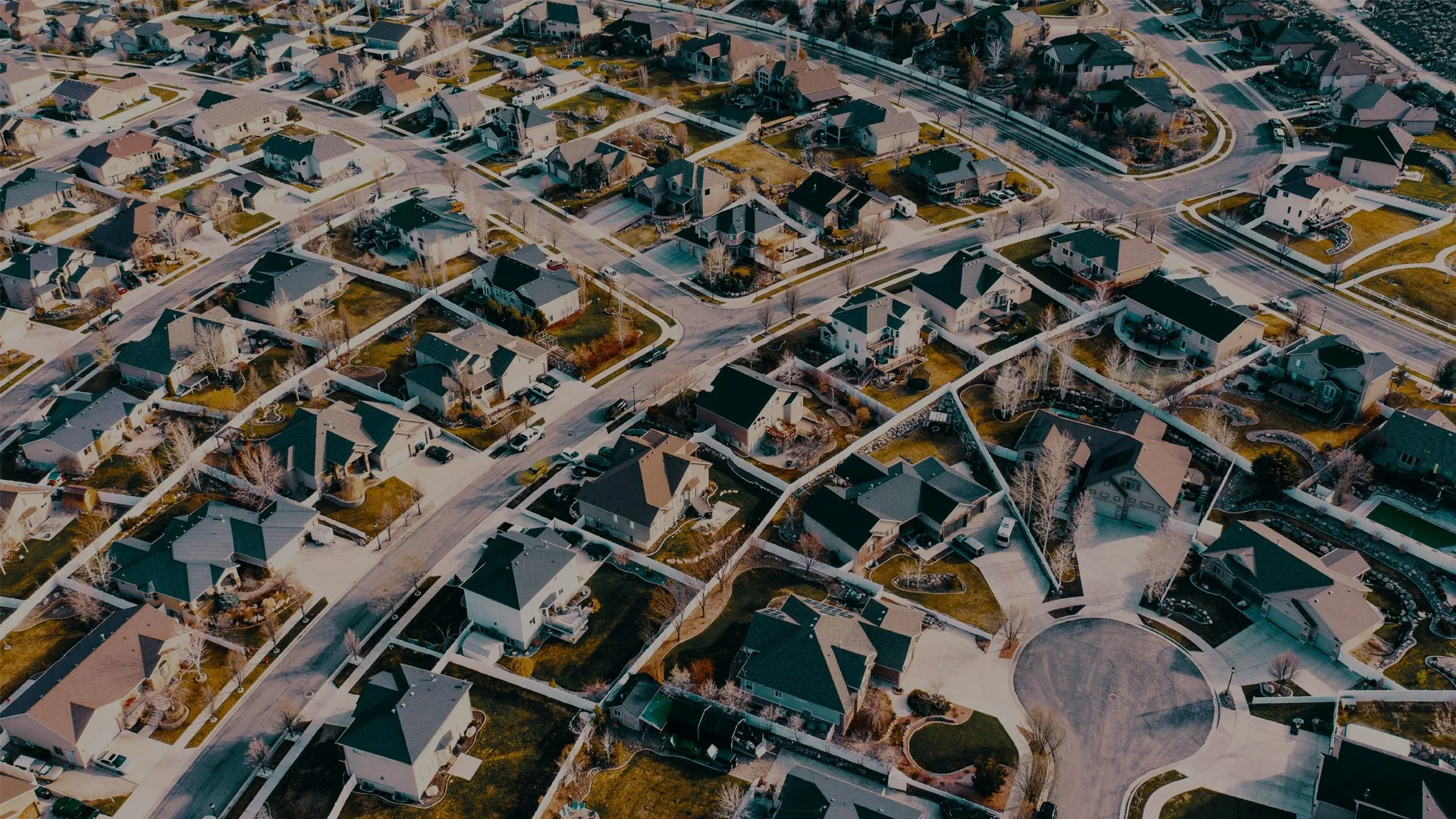
Predicting and mapping neighborhood-scale health outcomes: A machine learning approach
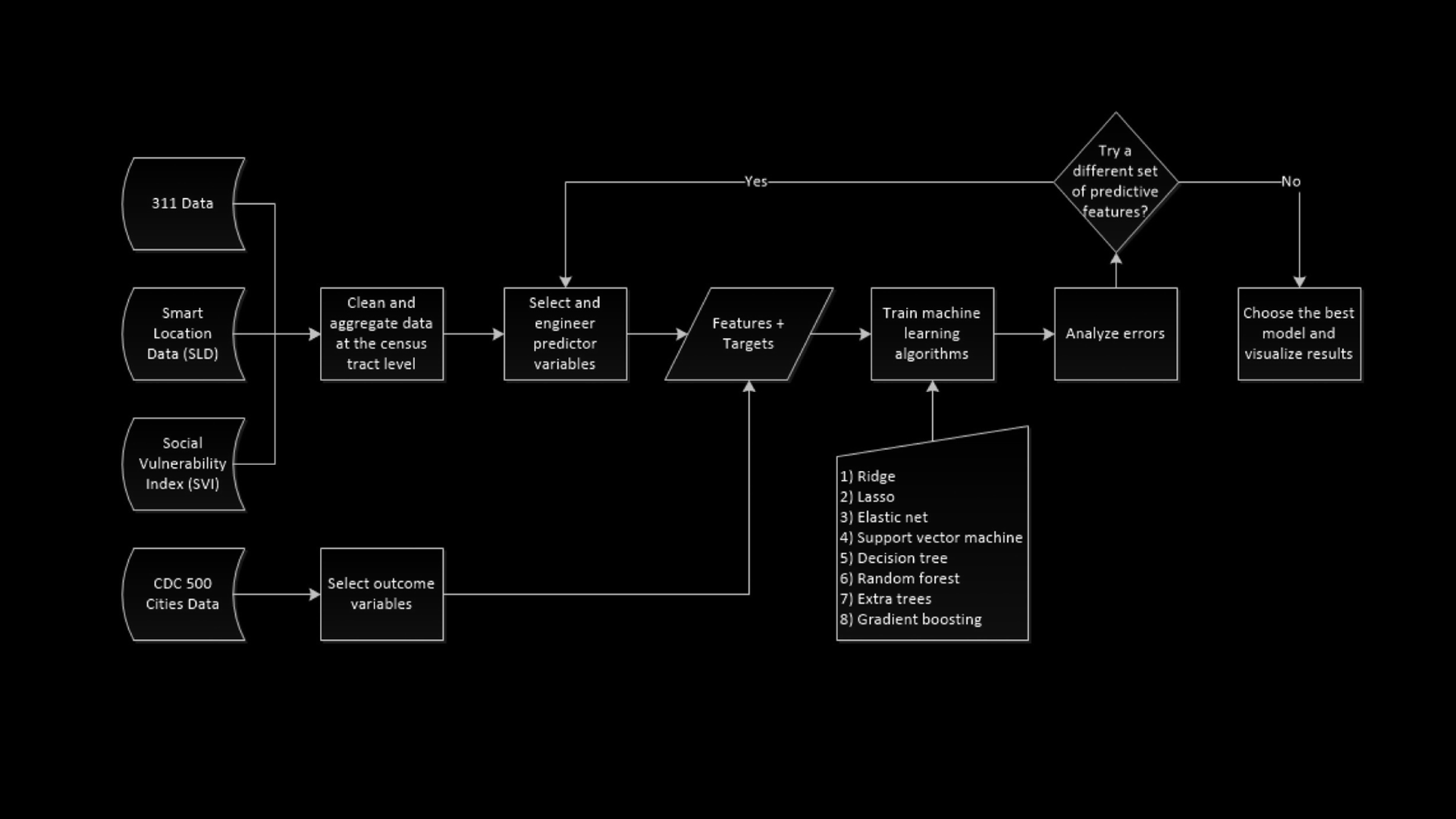
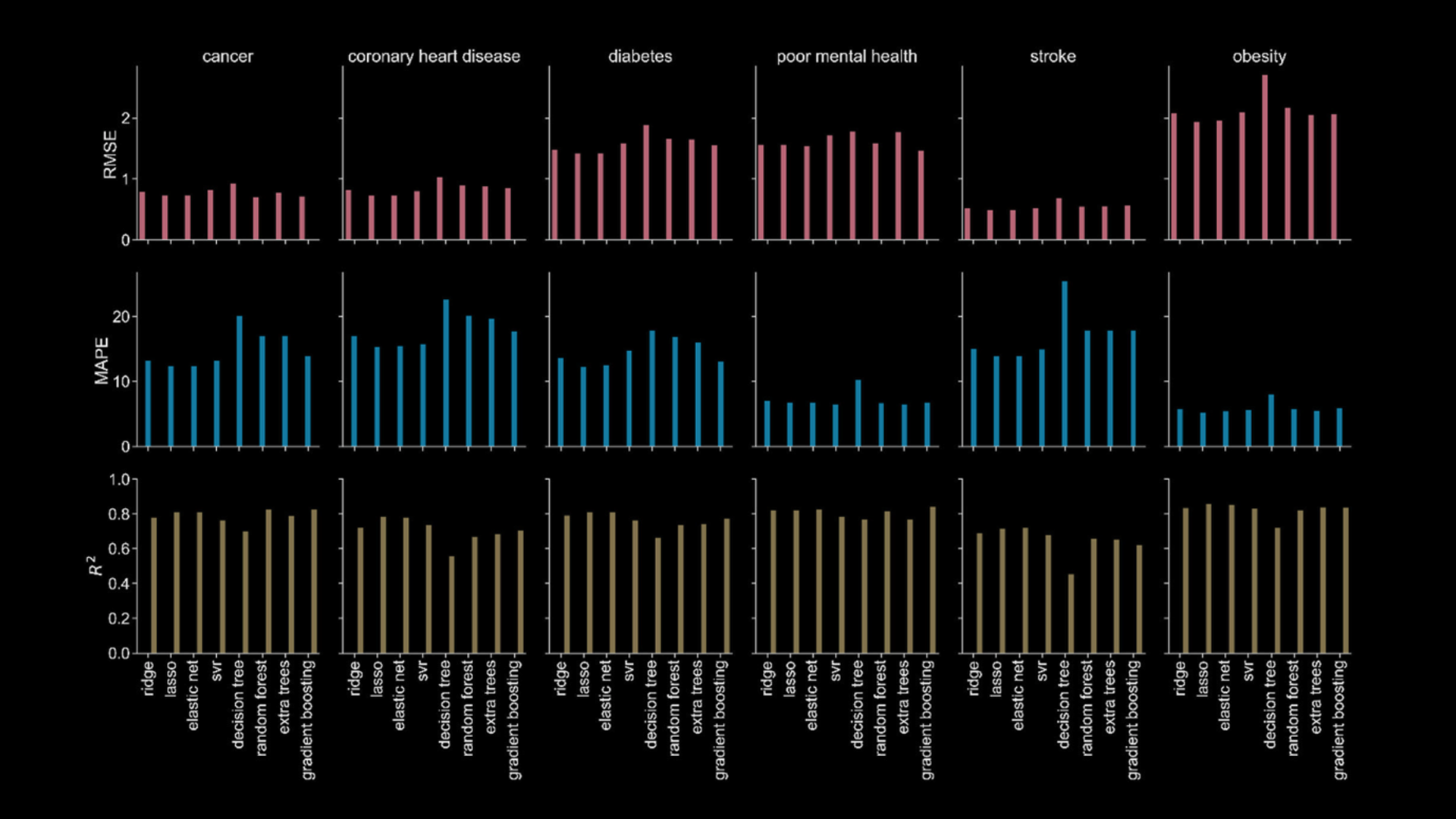

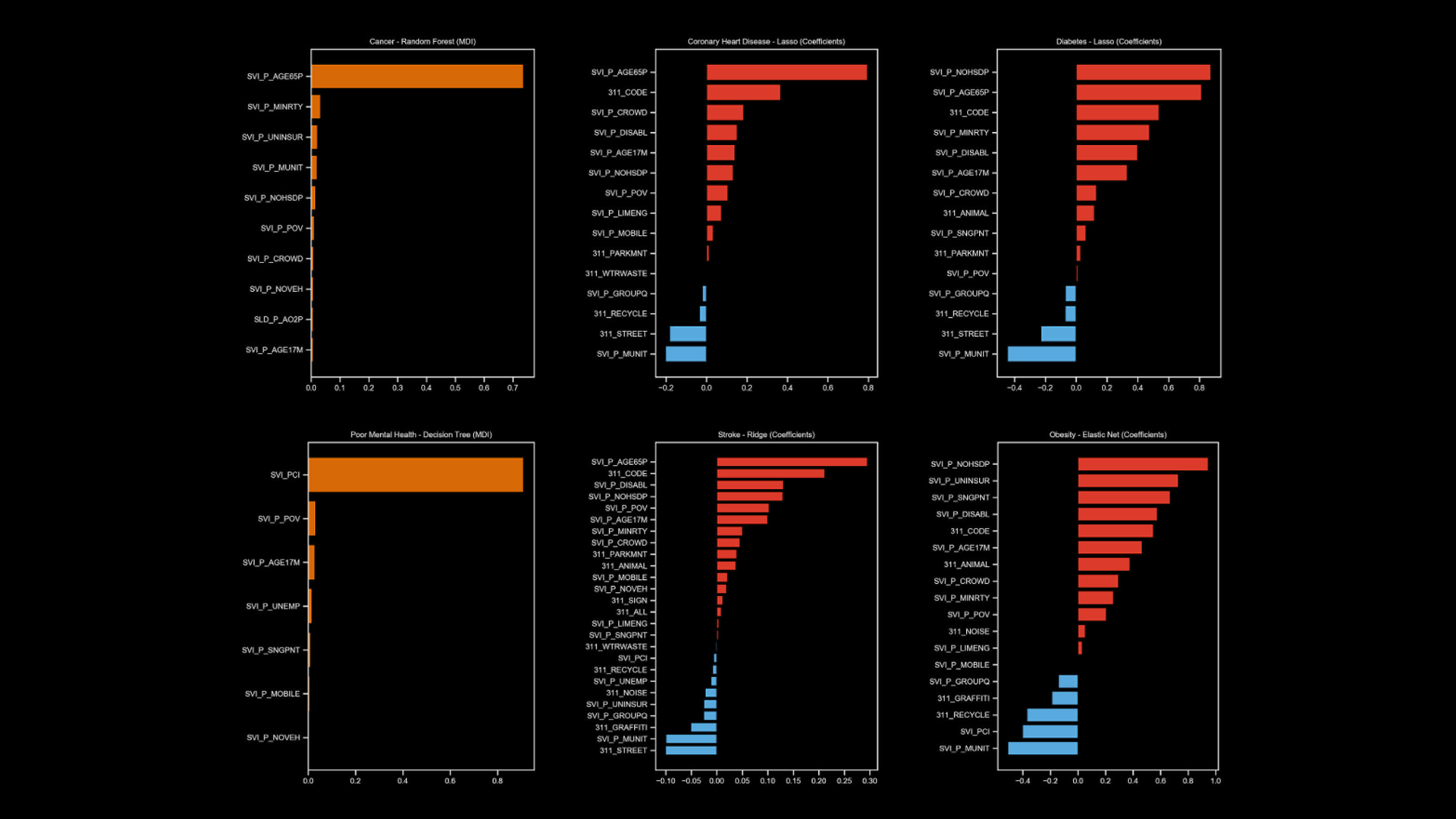
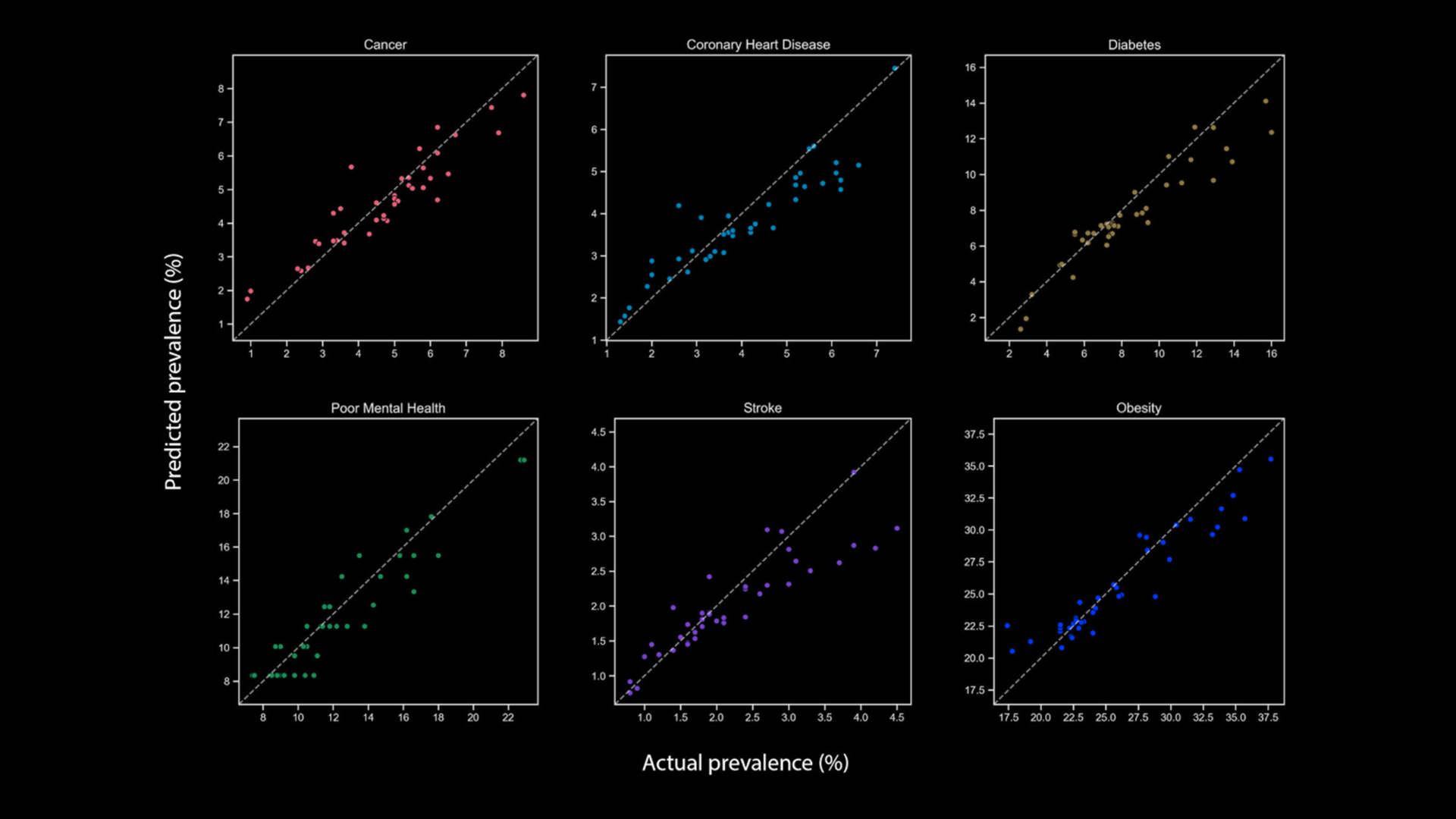

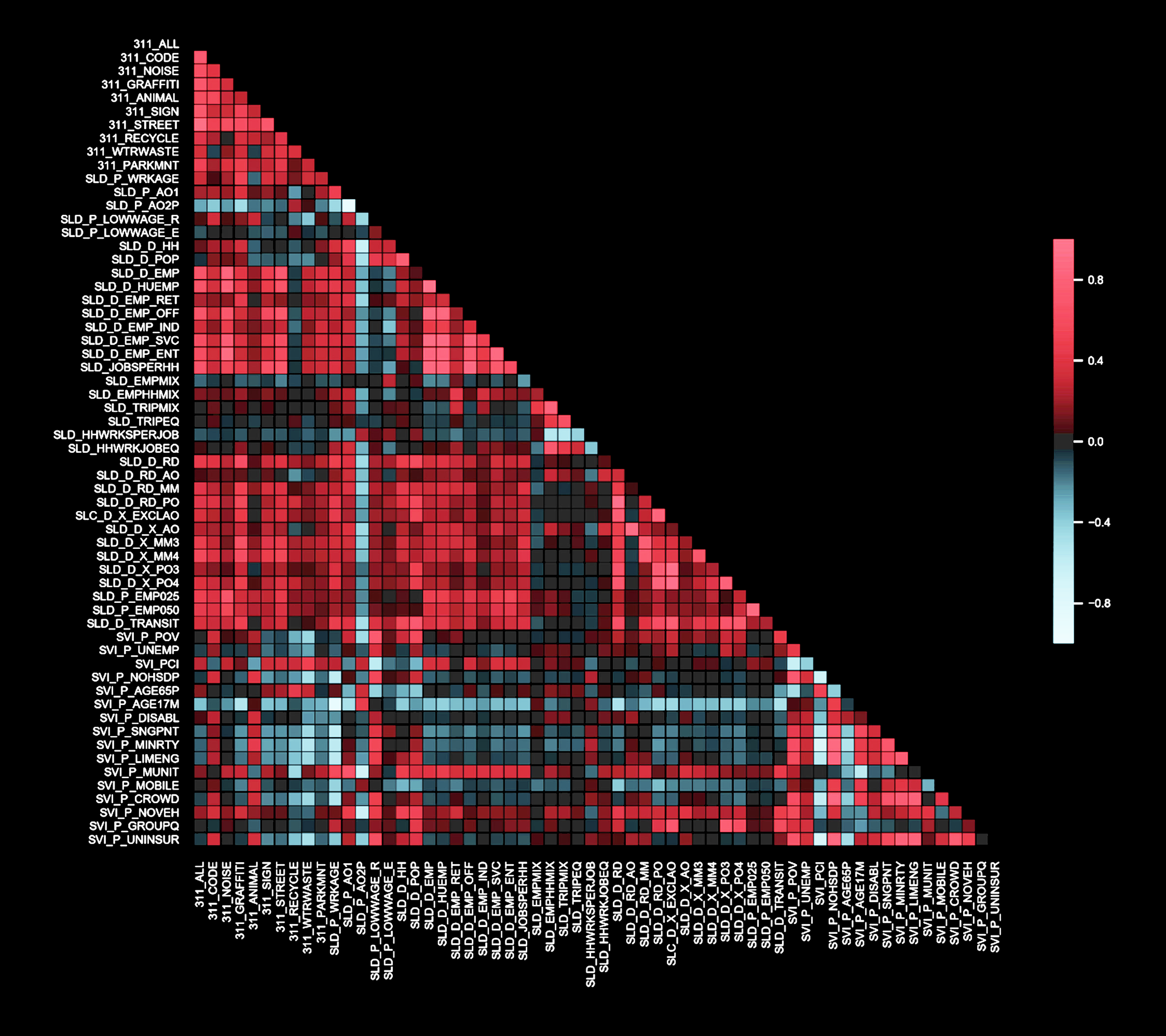
Abstract
Estimating health outcomes at a neighborhood level is crucial for urban health promotion but can be resource-intensive. This paper introduces a machine learning approach to predict the prevalence of six common chronic diseases at the census tract level in Austin, Texas. By experimenting with eight machine learning algorithms and 60 predictor variables, including social, physical environment, and neighborhood disorder factors, we found that sociodemographic and socioeconomic variables are the strongest predictors. Additionally, historical records of 311 service requests enhance model performance. These models can aid public health officials in assessing future scenarios and understanding how changes in neighborhood conditions affect health outcomes. Analyzing disparities between predicted and actual values can pinpoint areas for improvement or policy intervention.
Team
Chen Feng Junfeng Jiao